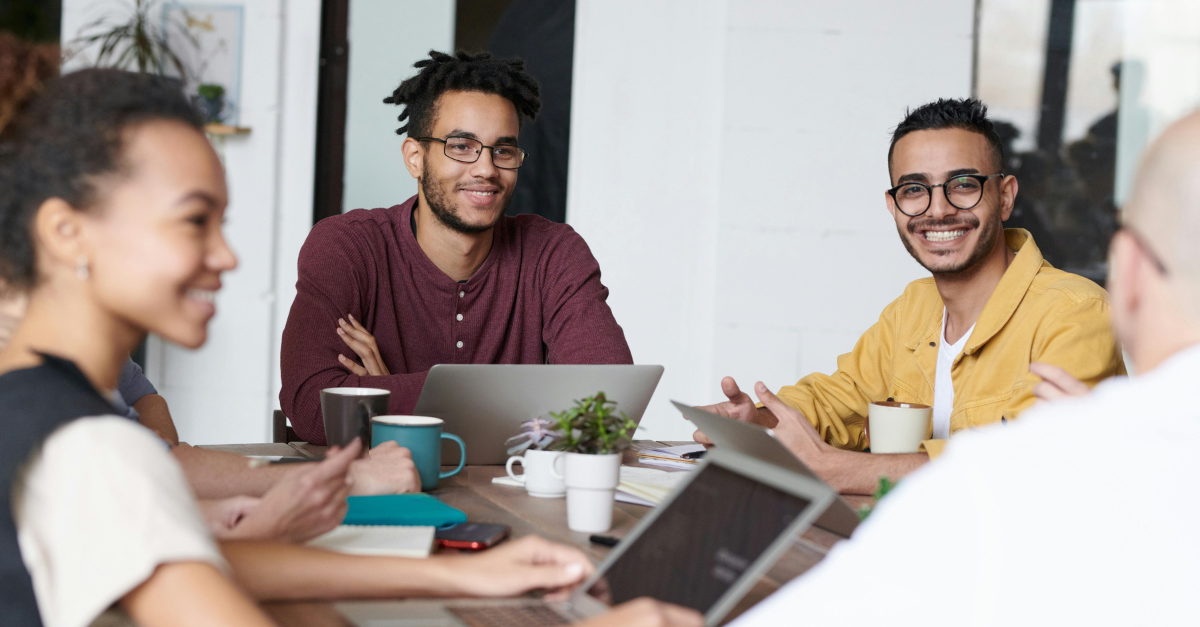
How we plan on using AI to find the perfect image for your campaign

We’ve set ourselves a challenge: can we use machine learning to reduce the time it takes to find the perfect image?
To quote the Norwegian playwright Henrik Ibsen, "a thousand words leave not the same deep impression as does a single deed", although author Tess Flanders put it more succinctly when she said: "Use a picture. It's worth a thousand words”.
With digital content constantly competing for our attention, this is true now more than ever. Which social post are you likely to notice and read - a dense wall of text or one with engaging pictures?
It’s not just about grabbing eyeballs. We know from our research into empathic marketing that images can prompt emotional reactions, which in turn, makes your audience more receptive to your message and the action you’d like to encourage.
Finding the right images for your content can be tricky
If images are so important, then marketing teams should put a lot of effort into finding the perfect ones to accompany their content. Surprisingly, this isn’t always the case. Images are often an afterthought, sourced at the last minute once the copy has been finalised. As any marketer knows, this can sometimes be a tedious task of hunting across shared network drives and stock libraries.
Even for teams with a decent Digital Asset Management (DAM) solution in place, finding the right image can take time. It requires experience and empathy. After all, you’re looking for an image that appeals to your audience, not just one that you like yourself.
Finding the perfect image for a new piece of content is essential but time-consuming - and that’s exactly the type of problem we like to solve at Bright! We’ve teamed up with Brighton-based machine learning experts BrightMinded to tackle this, on an R&D project we’ve codenamed KAPOW.
One of the first objectives of KAPOW is to understand the journey from when someone starts looking for an image for their new content to when they finally select the one that makes it into the published article. We’ll then use machine learning to see if we can shortcut this journey.
From there, we’ll try to improve the results, measure, learn and then repeat.
Existing AI features help, but they have their limits
Our DAM solution for smaller teams, Dash, already offers a AI-powered “search by content” feature. This allows content marketers to copy-and-paste their article into a popup text box to then see relevant images and videos.
Dash’s current search feature generates relevant keywords, which it then uses to scour the metadata of the photos and videos stored in a customer’s library.
For example, let’s take the first two paragraphs of one of our blogs earlier this year about the importance of safeguarding company culture during the coronavirus lockdown.
When we run this through Dash’s ‘search by content’ feature, the algorithm generates the following keywords: ‘behavioural sciences’, ‘psychology’, ‘action (philosophy)’, ‘human activities’, and ‘social psychology’.
In terms of keywords that describe the article text, that’s pretty decent. Depending on what you have in your Dash account, the images returned in a search using these keywords may even be suitable to use in the blog. But those keywords are of abstract concepts, which makes finding an image to accompany them difficult. When you then look at the images our content writer Emma selected for the blog, she chose photos of our staff relaxing at a company presentation and a collage of us on a Zoom call. How would our existing AI be able to make the associations that Emma was able to make in a split second? That’s just one of the challenges we’ll face when trying to automate this process.
For us to consider KAPOW a success, the new features we’ll develop should engage our users more effectively than Dash’s existing search features.
What if AI could teach itself when learning what blog images to feature?
Audiences value authenticity. They want to see images of people they relate to, rather than perfect-looking stock photography models, and they don’t like anything that looks hackneyed or cheesy. If you’re writing an article about the benefits of yoga and turn to sentiment analysis to find the image, it might bring up a picture of someone in the lotus position. It’s an obvious choice - too obvious. We reckon experienced content writers might settle on an image more tangential, like a relaxing mountain scene.
So that’s the challenge. By examining blog articles that already exist online, and the images that accompany them, can our machine learning model learn the relationship between text and the kind of images that an experienced human would choose for it?
That’s what we intend to find out. Watch this space!
Calling content creators: If you fancy helping us as we test this new feature, get in touch!
Dash is the perfect digital asset management solution for small teams. Try it yourself with a free, no-strings 14-day trial.